Sylvain Lardeau
Sylvain Lardeau
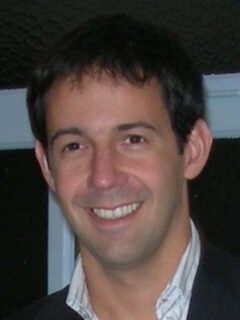
Siemens Digital Industries Software, Nürnberg, Germany
Performance, Potential and Pitfalls of Scale-Resolving Simulation in Industry, a Commercial CFD Perspective.
The widespread use of Computational Fluid Dynamic (CFD) software in industry is already quite well documented. The need to reduce development turn-around time while also providing increased insight into some physical aspects are the most common reasons mentioned. The inclusions of additional physics models (e.g. solid mechanics, electromagnetics) have also enabled to solve more and more complex problems, and the combination of CFD with adjoint and optimization methods is now used to reduce manufacturing and testing costs.
Statistical methods, such as Reynolds-Averaged Navier-Stokes (RANS) models, are still the most common turbulence modelling approach, and they will remain so in the industry for the foreseeable future. Those models are robust, they are mostly accurate in a large range of applications, their performance and limitations are now well-known by practitioners, and they are also compatible with a large range of other physics models.
On the other hand, a steady state is not suited to all flow problems. Unsteadiness can be introduced either geometrically, with solid body motion, or more likely by large-scale, non-turbulent, flow motion, like Von-Karman vortices. In these circumstances, RANS becomes inadequate, and one must thus turn to unsteady, scale-resolving (SRS) simulations. Examples range from simple external automotive aerodynamics (e.g., with the recent change of regulations, such as WLTP) to more complex cases like aeroacoustics, water management, etc.
This has contributed to a rise in a few industrial applications that are now routinely using SRS, not only for research purposes, as was previously the case, but also for day-to-day production practices. The democratization of SRS has been further enabled by a recent shift in computer chip designs, with new types of architecture such as those use in GPGPU, that are at least an order of magnitude faster than classical CPU, while being also well adapted to CFD algorithms. Modern programming languages and the most recent compilers also allow computation across various platforms using a unique source code, something that only the most recent CFD code can achieve, and this, in turn, leads to shorter turn-around computational time, both for steady and unsteady simulations.
SRS has some limitations, some inherent to the methods, and others that are due to the lack of reliable best practices. Setting up an SRS is still more prone to user error than an equivalent RANS setup. Resolution requirements can be unclear in complex problems, and so are the prescription of adequate boundary conditions. It must also be emphasized that, unlike popular belief, results are not always improved compared to classical steady-state RANS models. Other limitations of SRS are linked to the lack of (realistic) adjoint capabilities, and the handling of complex geometries, which already require complex and expensive CAD/meshing pipeline for RANS, can be even more problematic for SRS, with the additional requirement to resolve specific flow scales. This problem remains even when considering adaptative meshing. Not all is gloom, however. Recent projects about the use of AI in CFD have also shown how SRS could contribute to the further development of statistical models. A smart combination of steady-state and SRS seems to still represent the best compromise between speed, accuracy, and robustness, but this aspect has not received, so far, a lot of attention.
During the presentation, we will go through some examples ranging from some simple cases (e.g. cylinder flow) to very complex multi-physics configurations, and we will highlight the positive and negative aspects of each method. We will go into a bit more detail about the various turbulence models that are used in commercial CFD, their benefits and limitations, and where we think that progress can be achieved. We will also show how SRS can be used as part of a larger arsenal of tools used in a couple of industrial CFD applications.
Sylvain Lardeau is currently working in the CFD team for Siemens Industry Software, where he is the lead developer for turbulence and aeroacoustics for the commercial CFD software Simcenter STAR-CCM+. Prior to that, he was manager of the Wing Technology Center at Imperial College London, for 6 years, also teaching aerodynamic and turbulence courses at undergraduate and post-graduate levels. Beforehand, he spent some time working at PSA Peugeot-Citroen, Imperial College, after finishíng his PhD at the University of Poitiers.